Does the scientific process always begin with a question, or can it sometimes be sparked by a hunch, an observation, or a feeling that something isn't quite right? At the heart of scientific inquiry lies the hypothesis, a powerful tool that guides the investigation and shapes our understanding of the world.
The quest for knowledge is an intricate journey, often starting with curiosity. Sometimes, that curiosity crystallizes into a specific question, a desire to understand why something happens the way it does. However, the initial spark can also be more nebulous a suspicion, a pattern noticed in data, or a novel idea that seems to defy conventional wisdom. Regardless of the genesis, the next crucial step involves formulating a hypothesis. In essence, a hypothesis is a testable explanation for an observation or phenomenon. It's a tentative answer to a question, a proposed solution that can be investigated through experimentation and analysis.
The beauty of a hypothesis lies in its falsifiability. A good hypothesis isn't just a guess; it's a statement that can be proven wrong. It's designed in a way that allows scientists to gather evidence that either supports or refutes it. This is where the scientific method truly shines. The formulation of a hypothesis allows researchers to design experiments, collect data, and analyze the results to assess whether the evidence aligns with the proposed explanation. If the data consistently supports the hypothesis, it gains strength and may contribute to broader scientific theories. If the data contradicts it, the hypothesis is either revised or discarded, paving the way for a new line of investigation. The cycle of questioning, hypothesizing, testing, and refining is the cornerstone of scientific progress.
- All Star Riverhead Your Ultimate Guide To Bowling Fun
- Amber Marshall Photos Explore The World Of Heartland More
Now, let's delve into some key aspects of hypothesis testing and the critical role it plays in various fields of study. One of the fundamental concepts is the distinction between the null hypothesis and the alternative hypothesis. The null hypothesis (often denoted as H0) represents the default assumptionthe idea that there is no significant effect or relationship between the variables being studied. It's the starting point of the investigation. The alternative hypothesis (often denoted as H1 or Ha), on the other hand, proposes that there is a significant effect or relationship, and its the one that the researcher is typically trying to support. Formulating these two hypotheses is the crucial first step in hypothesis testing.
Once the hypotheses are established, the next stage involves selecting the appropriate statistical test. The choice of test depends on various factors, including the type of data collected (e.g., categorical, continuous), the number of groups being compared, and the research question. Some common statistical tests include t-tests, which are used to compare the means of two groups, and chi-square tests, which are used to analyze categorical data. Statistical tests calculate a test statistic, a value that summarizes the evidence against the null hypothesis. The test statistic is then used to calculate a p-value, which represents the probability of obtaining the observed results (or more extreme results) if the null hypothesis were true. If the p-value is below a predetermined significance level (usually 0.05), the null hypothesis is rejected, and the alternative hypothesis is supported. This doesn't necessarily mean the alternative hypothesis is definitely true, but the evidence suggests it is more likely than the null.
The significance level (alpha) is another crucial element. It is the threshold used to decide whether to reject the null hypothesis. Choosing a significance level, the researcher must acknowledge the possibility of making an error in their decision. There are two types of potential errors in hypothesis testing: Type I errors and Type II errors. A Type I error (or false positive) occurs when the null hypothesis is incorrectly rejected, concluding that there is an effect when there isn't one. The probability of committing a Type I error is equal to the significance level (alpha). A Type II error (or false negative) happens when the null hypothesis is incorrectly accepted, concluding that there is no effect when there is one. The probability of committing a Type II error is denoted as beta, and the power of a test is 1 - beta, representing the probability of correctly rejecting the null hypothesis when it is false.
- Honoring 911 Powerful Memorial Tattoos That Never Forget
- Tukuk Tuk Bluetooth Lock Smart Secure Digital Locking
Consider the example of a drug trial. The null hypothesis might be that the drug has no effect on a particular illness. The alternative hypothesis could be that the drug is effective. During the trial, researchers gather data on patients who receive the drug and compare their outcomes to a control group that doesn't receive the drug (often a placebo). Using the data, they apply a statistical test to assess whether the observed difference between the groups is statistically significant. If the p-value is lower than the significance level (0.05, for example), the researchers reject the null hypothesis and conclude that the drug is effective. This doesn't mean the drug will work for everyone, but the data supports its effectiveness. Conversely, if the p-value is greater than 0.05, the researchers do not reject the null hypothesis. They conclude that there is insufficient evidence to support the drug's effectiveness, though this doesn't necessarily mean it is ineffective.
Hypothesis testing is also employed in fields such as psychology. Imagine a study investigating the "picture superiority effect" the idea that pictures are remembered better than words. A researcher might hypothesize that the enhanced recall results from conceptual processing of the pictures distinctive features rather than the semantic characteristics. To explore this, the researchers would design experiments, perhaps presenting participants with lists of words and images and testing their recall after a delay. Statistical tests would then be used to compare the accuracy of recall for words and images, assessing whether the data supports the hypothesis.
The type of data significantly influences the choice of statistical tests. If the data consist of continuous variables like height or weight, the researcher may use t-tests or analysis of variance (ANOVA) to compare means between groups. For categorical data, such as the gender of participants or their preference for one product over another, the chi-square test of independence is often suitable. Other factors come into play as well, such as the sample size; smaller samples can require a larger effect to be considered statistically significant. The distribution of the data, i.e., whether it is normally distributed or not, can determine the choice of parametric or non-parametric tests.
Understanding these elementsthe null and alternative hypotheses, the choice of statistical tests, significance levels, and the potential for erroris critical for sound scientific research. A well-formulated hypothesis and proper statistical analysis are the pillars for drawing meaningful conclusions from data and building reliable knowledge. Furthermore, it's crucial to remember that hypothesis testing is just a tool. It provides evidence for or against a hypothesis, but it's never definitive proof. The best scientific results come from a combination of rigorous methodology, insightful interpretation, and an understanding of the limitations of the chosen methods. As science progresses, our hypotheses become more refined, and our understanding of the world evolves.
The field of clinical judgment in healthcare relies heavily on hypothesis generation and testing. For instance, when a healthcare provider assesses a patient, they may consider a variety of signs and symptoms. Their initial observations, education, and past experiences guide the formation of a preliminary hypothesis (a potential diagnosis or cause of the patient's condition). They gather additional information from the patient, such as medical history, symptoms, and test results, which represents the process of synthesizing information.
Using critical thinking, the healthcare professional then evaluates this information, weighs the evidence for or against different possibilities, and refines their hypothesis. This iterative process involves evaluating evidence, identifying patterns, and making decisions about the most likely diagnosis and the most appropriate course of action. In this context, the "hypothesis" is a working diagnosis. Further, if the patient does not improve with the prescribed treatment, it suggests the need for further investigation, potentially leading to the testing of alternative diagnoses. Clinical judgment is a complex process of generating and testing hypotheses based on observations, experience, and knowledge, all to improve patient care.
Moreover, hypothesis generation isn't just a formal part of the scientific process. It is inherent in our everyday lives. Consider the classic example of someone trying to repair a broken appliance. They might form a hypothesis about the cause of the failure (e.g., a blown fuse or a disconnected wire). They would then perform actions to test the hypothesis (e.g., checking the fuse, examining the wiring). If those tests are successful, the hypothesis is confirmed and the problem is solved. If not, the person would generate a new hypothesis and repeat the testing process.
The world of language development offers another compelling area where hypotheses are formulated and tested. Research into how children learn language offers an intriguing avenue for investigating the processes involved. For instance, linguists might hypothesize that childrens use of modal verbs like "can" and "will" is based on their understanding of abstract concepts such as possibility and intention. This leads to experiments that involve observing language use and assessing how it aligns with the hypothesis. Furthermore, the way children construct sentences, and the order in which they acquire grammatical rules, contribute to a deeper comprehension of the complex phenomenon of human language acquisition.
In the world of scientific research, there's an emphasis on finding and downloading hypothesis pictures, using these as visual aids for projects, presentations, or educational resources. Images can enhance the understanding of complex concepts, but they are by no means a substitute for robust investigation, with the appropriate data analysis to support or refute a given hypothesis.
The path of scientific inquiry, therefore, is paved with hypotheses. They are the starting point for investigations, the anchors for experiments, and the guides for understanding. From the intricacies of language to the mysteries of the universe, the scientific method is a constant reminder that the process of inquiry is never truly over. Each confirmed hypothesis provides a step forward, while each refuted hypothesis inspires new questions and fresh exploration.
Example | Description |
---|---|
Picture Superiority Effect | The concept that pictures are remembered better than words. |
Conceptual Processing | Analysis that focuses on a picture's distinctive features. |
Semantic Features | Relates to the meaning or the conceptual understanding of the picture. |
Null Hypothesis | The hypothesis that there is no significant effect or relationship between the variables being studied. |
Alternative Hypothesis | The hypothesis that there is a significant effect or relationship. |
Statistical Tests | Methods like t-tests or chi-square tests, used to analyze data. |
P-value | The probability of obtaining observed results if the null hypothesis is true. |
Significance Level | The threshold (e.g., 0.05) used to decide whether to reject the null hypothesis. |
Type I Error | Rejecting the null hypothesis when it is actually true (false positive). |
Type II Error | Failing to reject the null hypothesis when it is false (false negative). |
This table clarifies important concepts related to hypothesis testing, offering concise descriptions for better comprehension and further study.

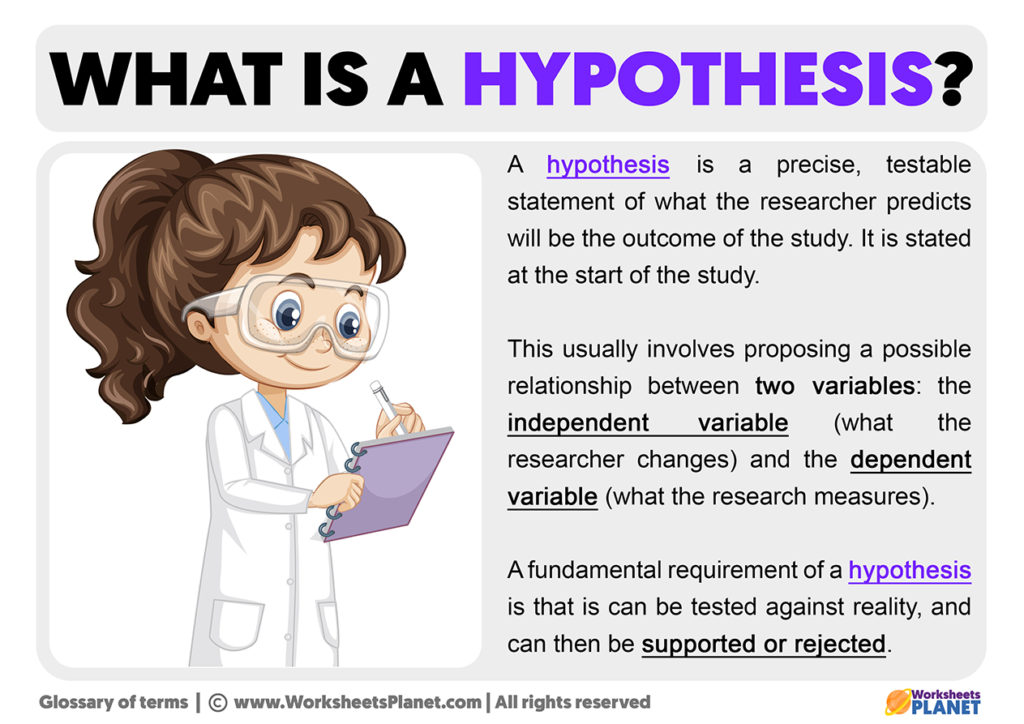

Detail Author:
- Name : Audrey Walter PhD
- Username : ullrich.kayley
- Email : jess90@wintheiser.com
- Birthdate : 2000-12-13
- Address : 494 Dell Orchard Apt. 097 Pacochafurt, MO 10683-6001
- Phone : +1-724-218-9936
- Company : Brown-Lesch
- Job : Home Health Aide
- Bio : Quia quos veritatis quibusdam nam qui et et. Enim corporis ut rerum numquam vitae iure. Voluptas dolores quos voluptas dolorem aliquam eos et.
Socials
twitter:
- url : https://twitter.com/mabelle_real
- username : mabelle_real
- bio : Quisquam nemo earum corporis suscipit temporibus. Vel cumque qui voluptatibus esse velit sint similique. Quibusdam voluptatem et et laudantium.
- followers : 4868
- following : 1259
tiktok:
- url : https://tiktok.com/@mabelle_dev
- username : mabelle_dev
- bio : Quibusdam qui nemo natus velit deserunt temporibus.
- followers : 5659
- following : 2446
linkedin:
- url : https://linkedin.com/in/mkrajcik
- username : mkrajcik
- bio : Minima harum qui nulla veniam error.
- followers : 1076
- following : 2845
facebook:
- url : https://facebook.com/mabelle3879
- username : mabelle3879
- bio : Officia quidem corrupti assumenda aperiam voluptatem inventore.
- followers : 5226
- following : 2033